AI for the Public Good
Despite ethical landmines, innovations in artificial intelligence can be leveraged to address society's problems.
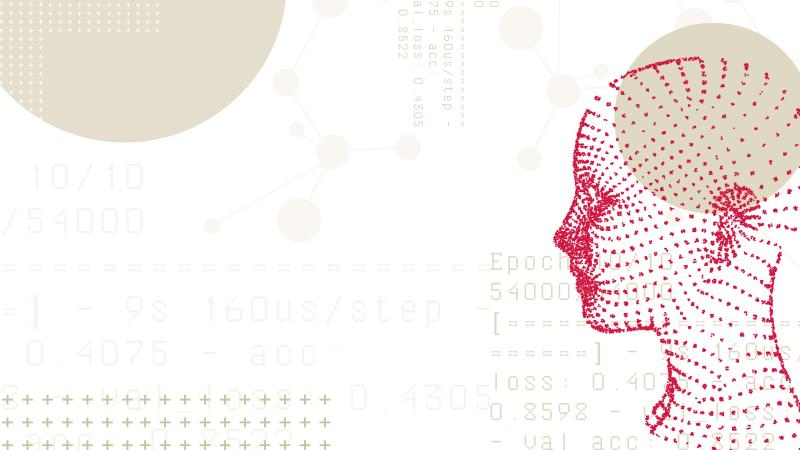
Following ChatGPT's was release to the public, a slurry of new artificial intelligence products—like computer programs that can write in the style of your favorite author and software that can generate images mimicking any artist’s brushstroke—exploded onto the scene, spurring fears and debates over how AI will shape the future of work, education, arts and culture, and nearly every other aspect of our lives.
Originating in the 1950s, AI is not a new concept, says Kerstin Haring, assistant professor of computer science in the Daniel Felix Ritchie School of Engineering and Computer Science. “The math was solved a long time ago,” Haring says. “But we needed the computational power. And for a long time, we didn’t have the amount of data necessary to make these large models work.”
And while consumer-facing products and services like ChatGPT feel like shockingly new inventions, the underlying technology has played a behind-the-scenes role in our daily lives for years. Everything from credit card fraud monitoring and airline ticket pricing to Netflix recommendations and social media feeds are powered by AI, says Stephen Haag, professor of the practice in the Daniels College of Business.
Self-driving cars, AI image generators, bookkeeping and other AI-driven software suites are just the first stage of services enabled by recent improvements in computing power and data infrastructure. Though scary for some, recent developments in AI provide a suite of new tools for researchers across disciplines.
Ethics and bias in machine learning
Artificial intelligence describes a broad set of fields, but machine learning—computer programs that can recognize patterns in data, then build statistical models and find patterns in other data, make predictions or generate new data accordingly—is the most prominent. In her previous research, Haring and fellow researchers developed Build-A-Bot, an interactive platform that lets users design robots. Haring is planning to train a machine learning system on data from the user-generated designs to build robots that humans can more comfortably and efficiently recognize and interact with.
There are different approaches to training AI: supervised, with humans assisting an AI system to recognize patterns; reinforcement, with an AI system being scored on how right or wrong it is; and unsupervised, where the AI system is given huge amounts of data to process on its own. All three vary in their function and their purpose, but according to Haring, they share serious ethical implications if not designed carefully. “It’s hard to retrofit ethics into a system,” she says.
And for a computer, recognizing a new pattern is no easy task, requiring massive amounts of data to train the system on.
Data used for training is often scraped from publicly accessible web pages or acquired without the knowledge of copyright holders, leading to AI-generated text and images that bear a striking resemblance to the manmade works they were trained on, raising questions about theft and copyright violations. While litigation is underway—and more will certainly follow—there are yet to be substantive regulatory or legislative guidelines on AI data sources. And, Haring adds, AI is trained to recognize and reflect patterns from real world data, raising further ethical concerns. “We live in a biased system, so the data that we create is already biased,” Haring says. “By learning the patterns in that data, it can perpetuate and reinforce certain biases—which is a problem.”
Like any technology, AI does not exist in a vacuum. Navigating AI’s complex and ever-changing ethical landscape requires diverse, interdisciplinary teams of researchers, developers and users alike to consider where training data comes from, what societal patterns will be reflected in the data and the effects that an AI’s implementation has on real people.
Fears that AI will destroy the world and end life as we know it are, fortunately, not totally realistic—at least not with existing technological infrastructure, Haag says. But AI will have significant impacts on education, work, transportation, medicine and most other fields.
“I look at it from three points of view: efficiency, effectiveness and innovation. And I think it’s innovation in the space that has a lot of people really frightened,” he says. “We’re going see a transition in a lot of job areas where AI is going to be able to take over some aspects of it, but not all,” he says.
And for Haag, the potential for AI-enabled smart homes, tailor-made educational software and breakthroughs in health care and medical research—like DU researchers’ ongoing application of AI to air pollution, infectious disease and substance use interventions—is far more exciting than worrisome.
Modeling real-time air quality
From respiratory diseases to cardiovascular issues and premature death, exposure to fine pollutant particles in the air has serious consequences. While air quality is tracked by organizations including the Environmental Protection Agency, the data often covers wide areas and is updated daily, at best, making it challenging for vulnerable populations to protect themselves as air quality fluctuates from neighborhood to neighborhood from hour to hour. Recently, Jing Li, associate professor in the Department of Geography and the Environment, used machine learning to develop a precise, real-time system to track and predict air quality across the Denver metro area.
Combining a network of low-cost air quality sensors with machine learning techniques including graph embedding to track spatial data; long short-term memory to account for time; and neural networks to integrate environmental and societal factors, the system allows for considerably more up-to-date tracking and prediction of air quality and is far more geographically precise. Its impacts are immediate. “Predicting PM2.5 concentrations helps individuals—particularly those with respiratory diseases or long COVID— take precautions to reduce exposure, ultimately leading to better health outcomes,” she says. In her previous research, Li also developed a model simulating the spread of COVID-19 among neighborhoods.
Youth substance use and homelessness interventions
In addition to tracking and predicting environmental and biological events, DU researchers are finding that AI can be useful in tackling social problems. Anamika Barman-Adhikari, associate professor in the Graduate School of Social Work, developed a machine learning system that assists social workers in designing group drug-use interventions for youth experiencing homelessness.
Such interventions are typically made up of peer-led groups that are randomly assigned and, at times, result in deviancy training—when individuals learn and reproduce harmful behaviors from their peers. By mapping individuals’ networks of relationships and behaviors, Barman-Adhikari’s AI system simulated the outcomes for each potential group configuration and selected the group with the best outcomes. The AIassisted groups showed nearly a 60% reduction in deviancy training over the randomly assigned groups.
Barman-Adhikari previously used AI to predict substance use based on conversations and posts from Facebook feeds. The AI-powered algorithm was 80% accurate in predicting an individual’s substance use compared to approximately 30% using traditional statistical models. For Barman-Adhikari, AI is shaping up to be a tool for triage, allowing researchers and social workers to maximize the effectiveness of the limited resources available to them.
With promising results from this and other recent research, Barman-Adhikari says that if researchers and policymakers heed caution in the development and use of new AI tools, they will be better able to avoid reproducing harmful societal biases and other consequences. “Some of the fears are legitimate,” she says. “I think we need more awareness of what the technology can do. We also need to advocate for better regulation. But the silver lining is that if this technology is used wisely, I think it can radically change our lives for the better.”
This article was adapted from the Winter 2024 issue of the University of Denver Magazine. Visit our website to read the rest of the stories in this issue.